英语翻译CS techniques are appropriate for monostatic,bistatic,an
来源:学生作业帮 编辑:大师作文网作业帮 分类:英语作业 时间:2024/11/14 12:47:44
英语翻译
CS techniques are appropriate for monostatic,bistatic,and multiscatic (many receivers and transmitters) scenarios.Since the radar receiver is greatly simplified,CS provides a powerful yet inexpensive framework for multistatic sensor network radars,where the radar signals resulting from one transmitting antenna are received at many receiving antennas that,by virtue of our simplifications,canbe made very simply and inexpensively.Our results can also be combined with the theory of distributed compressed sensing (DCS) [11],[12] for array processing and beamforming type applications.The CS-based imaging framework introduced here also applies directly to other modalities such as sonar and synthetic aperture sonar imaging.
While the CS literature has focused almost exclusively on problems in signal reconstruction,approximation,and estimation,CS is information scalable to a much wider range of statistical inference tasks.Detection,classification,and recognition do not require a reconstruction of the signal,but only require estimates of the relevant sufficient statistic for the problem at hand [13].A key point is that it is possible to directly extract these statistics from a small number of random measurements without ever reconstructing the signal.The two upshots are that significantly fewer measurements can be required for signal detection than for signal reconstruction and that the computational complexity of detection can be much reduced compared to reconstruction.Both of these bode well for radar applications,since if we are merely interested in detecting targets rather than reconstructing images of them,then we can use an even lower sampling rate for the CS based receiver.Moreover,in many radar applications,target detection,classification,and recognition decisions are often made based on the result of some kind of matched filtering or correlation with a set of templates.Information scalability enables us to compute close approximations to these matched filter results directly from incoherent measurements without having to perform expensive reconstruction or approximation computations [14].
There are a number of challenges to be overcome before an actual CS-based radar system will become a reality.First,the target reflectivity being probed must be compressible in some basis,frame,or dictionary.Second,the signal recovery algorithms
must be able to handle real-world radar acquisition scenarios with sufficient computational efficiency and robust performance for noisy data.Third,there is a subtle tradeoff to optimize between the reduction in sampling rate \7N/M\x08 and the dynamic range
of the resulting CS system [15].These are areas of active research for both our team and the broader CS community.In particular,there could be links with recent work on finite rate of innovation sampling for ultrawideband communication systems [16].
CS techniques are appropriate for monostatic,bistatic,and multiscatic (many receivers and transmitters) scenarios.Since the radar receiver is greatly simplified,CS provides a powerful yet inexpensive framework for multistatic sensor network radars,where the radar signals resulting from one transmitting antenna are received at many receiving antennas that,by virtue of our simplifications,canbe made very simply and inexpensively.Our results can also be combined with the theory of distributed compressed sensing (DCS) [11],[12] for array processing and beamforming type applications.The CS-based imaging framework introduced here also applies directly to other modalities such as sonar and synthetic aperture sonar imaging.
While the CS literature has focused almost exclusively on problems in signal reconstruction,approximation,and estimation,CS is information scalable to a much wider range of statistical inference tasks.Detection,classification,and recognition do not require a reconstruction of the signal,but only require estimates of the relevant sufficient statistic for the problem at hand [13].A key point is that it is possible to directly extract these statistics from a small number of random measurements without ever reconstructing the signal.The two upshots are that significantly fewer measurements can be required for signal detection than for signal reconstruction and that the computational complexity of detection can be much reduced compared to reconstruction.Both of these bode well for radar applications,since if we are merely interested in detecting targets rather than reconstructing images of them,then we can use an even lower sampling rate for the CS based receiver.Moreover,in many radar applications,target detection,classification,and recognition decisions are often made based on the result of some kind of matched filtering or correlation with a set of templates.Information scalability enables us to compute close approximations to these matched filter results directly from incoherent measurements without having to perform expensive reconstruction or approximation computations [14].
There are a number of challenges to be overcome before an actual CS-based radar system will become a reality.First,the target reflectivity being probed must be compressible in some basis,frame,or dictionary.Second,the signal recovery algorithms
must be able to handle real-world radar acquisition scenarios with sufficient computational efficiency and robust performance for noisy data.Third,there is a subtle tradeoff to optimize between the reduction in sampling rate \7N/M\x08 and the dynamic range
of the resulting CS system [15].These are areas of active research for both our team and the broader CS community.In particular,there could be links with recent work on finite rate of innovation sampling for ultrawideband communication systems [16].
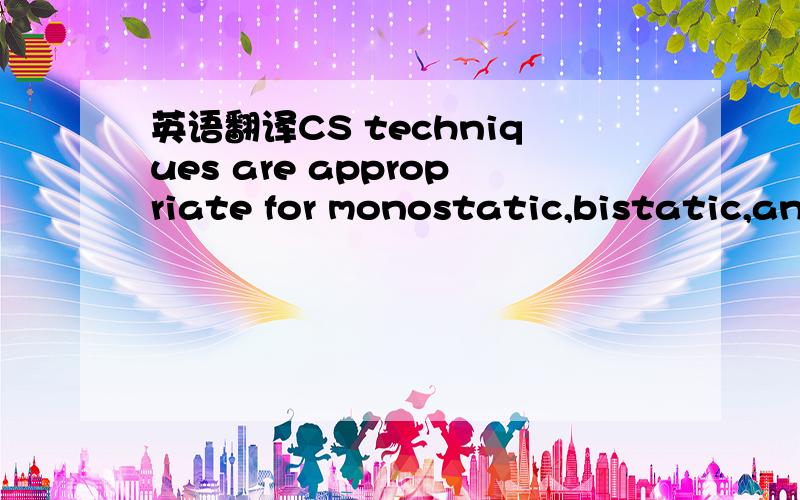
CS技术可以应用到单基,双基,多基雷达(带有多套收发器).由于极大简化了雷达接收器,CS技术为多基感测网路雷达提供了威力大且成本不高的框架,一处发送天线的信号能够被多处接收天线接受,通过简化设计,不仅操作简便,而且降低了成本.可以把我们的研究成果与分散式压缩传感理论相结合,应用到阵列处理和波束成形.这里介绍的基于CS技术的成像框架还可以直接应用到声纳与合成孔径声纳成像系统.
当前大多关于CS技术的文献都专注于信号的重构,近似值,估算,但CS技术可以应用到更广泛的数据推测任务当中.探测,分类,识别不需要信号的重构,只需得到足够的相关估算数据,就可以解决问题.关键是我们可以从少量随机计算中直接挖掘这些数据,而无须对信号进行重构.这样会得到两个结果,一是信号探测比信号重构所需的计算可以大量减少;二是与信息重构相比,可以极大减少计算的复杂性.这些都有利于雷达的应用,因为如果我们只专注探测目标,而不是图像重构,那么基于CS技术的接收器就可以使用更低的采样率.
而且,许多雷达的应用,对目标的探测,分类,识别都是建立在某类匹配滤波结果之上,或与模版相关联.信息的可拓展性使我们能够计算直接来源于不连贯测量值的匹配滤波的近似值,而不必进行昂贵的重构或近似值计算.
我们仍需要逾越许多挑战,才能开发出真正的基于CS技术的雷达系统.首先,探测的目标反射率必须能够在某些基,框架或代码词典中压缩;第二,信号恢复算法应用在真实的雷达获取目标时,针对噪音数据必须具备足够的计算效率和强劲性能.第三,需要在减少采样率和CS系统的动态范围之间达到微妙的平衡.以上是我们这个团队以及整个CS技术团体积极开展的研究领域,这可能与最近为超宽带通讯系统开展的关于创新采样有限率的研究有关.
当前大多关于CS技术的文献都专注于信号的重构,近似值,估算,但CS技术可以应用到更广泛的数据推测任务当中.探测,分类,识别不需要信号的重构,只需得到足够的相关估算数据,就可以解决问题.关键是我们可以从少量随机计算中直接挖掘这些数据,而无须对信号进行重构.这样会得到两个结果,一是信号探测比信号重构所需的计算可以大量减少;二是与信息重构相比,可以极大减少计算的复杂性.这些都有利于雷达的应用,因为如果我们只专注探测目标,而不是图像重构,那么基于CS技术的接收器就可以使用更低的采样率.
而且,许多雷达的应用,对目标的探测,分类,识别都是建立在某类匹配滤波结果之上,或与模版相关联.信息的可拓展性使我们能够计算直接来源于不连贯测量值的匹配滤波的近似值,而不必进行昂贵的重构或近似值计算.
我们仍需要逾越许多挑战,才能开发出真正的基于CS技术的雷达系统.首先,探测的目标反射率必须能够在某些基,框架或代码词典中压缩;第二,信号恢复算法应用在真实的雷达获取目标时,针对噪音数据必须具备足够的计算效率和强劲性能.第三,需要在减少采样率和CS系统的动态范围之间达到微妙的平衡.以上是我们这个团队以及整个CS技术团体积极开展的研究领域,这可能与最近为超宽带通讯系统开展的关于创新采样有限率的研究有关.
英语翻译Using techniques first developed for the offshore oil an
英语翻译The search for an appropriate “graphic density”13 isesse
英语翻译It is clearly not appropriate for a student to litter an
英语翻译The techniques for welding titanium are,have to be actua
What would be appropriate clothes to wear for an interview?
英语翻译useing techniques developed for the offshore oil and gas
英语翻译Important methodological issues and techniques for elect
英语翻译appropriate criteria includes notification of an issue t
英语翻译Identify an appropriate framework in which to evaluate t
英语翻译Apply an appropriate amount to moist face after washing.
英语翻译he appropriate working standard solutions for fortificat
what are appropriate topics for 'small talk',and what are in