英语翻译Inthissection wewillprovidea formaldefinitionof th
来源:学生作业帮 编辑:大师作文网作业帮 分类:英语作业 时间:2024/11/13 20:01:41
英语翻译
Inthissection wewillprovidea formaldefinitionof the clus- teringproblemandintroduceanotationusedintherestofthe paper.Where super- or subscripts are used,we will reuse i,j,l,m.No meaning is implied where these are carried over between definitions.As is customary in literature,bold-faced variables indicate sets,with|Y|defined as the number of el- ements in set Y,i.e.,its size.A n-dimensional feature or attribute vector x = (x1,x2,...,xn) is called a data object,with xi,(i = 1,2,...,n) the i-th feature,attribute,or data value.A dataset X = {x1 ,x2,...,xN} has N = |X| data objects,with xi,(i = 1,2,...,N) the i-th data object.A clustering C is a col- lection of data object subsets ci (i = 1,2,...,k),called clusters,with k = |C| traditionally reserved to denote the size of the clustering; the number of clusters,|c|denotes the sizeofcluster c,i.e.,thenumberofdataobjectsinthecluster.A cluster is not allowed to be empty:c 6= ∅ or |c| 6= 0,and the conjunction of all clusters contains all data objects of the dataset:c1 ∪c2 ∪...∪ck = X.In a non-overlapping clustering,all clusters are mutually disjunct:ci ∩ cj = ∅,for i 6= j.If the mutual disjunction criteria is relaxed,clus- tering C is said to be overlapping.Here we only consider non-overlapping clustering.The Euclidean distance metric d(xi,xj) is used to cal- culate the distance between two data objects xi and xj.d(x,c) is used to indicate the set of distances between data object x and all data objects in cluster c:d(x,c) = {d(x,x0 1),d(x,x0 2),...,d(x,x0 |c| )} with x0 ∈ c.The inner-cluster distance of cluster c is used as the basis for the fitness function and most of the heuristics,and is calculated as fol-
lows:
D(c) =
|c|−1 X l=1
|c| Xm =l+1
d(xl,xm) (1)
where xl ∈ c and xm ∈ c.If the inner-cluster distance is calculated for a clustering C,it produces a set contain- ingtheinner-clusterdistancesofallclustersintheclustering:D(C) = {D(c1),D(c2),...,D(ck)}.Theinner-clusterdis- tance of data object x is defined as its contribution to the inner-cluster distance of the cluster it belong to:D(x) = P|c| i=1 d(x,xi) where x ∈ c and xi ∈ c.If the inner-cluster distance of a dataset X is calculated,it produces a set con- taining the inner-cluster distances of all data objects in the dataset:D(X) = {D(x1),D(x2),...,D(xN)).The fitness functionusedtoevaluateclusteringCisthecumulativeinner- cluster distance of the clustering:
f(C) =
k X i=1
D(ci) (2)
where ci ∈ C.
Inthissection wewillprovidea formaldefinitionof the clus- teringproblemandintroduceanotationusedintherestofthe paper.Where super- or subscripts are used,we will reuse i,j,l,m.No meaning is implied where these are carried over between definitions.As is customary in literature,bold-faced variables indicate sets,with|Y|defined as the number of el- ements in set Y,i.e.,its size.A n-dimensional feature or attribute vector x = (x1,x2,...,xn) is called a data object,with xi,(i = 1,2,...,n) the i-th feature,attribute,or data value.A dataset X = {x1 ,x2,...,xN} has N = |X| data objects,with xi,(i = 1,2,...,N) the i-th data object.A clustering C is a col- lection of data object subsets ci (i = 1,2,...,k),called clusters,with k = |C| traditionally reserved to denote the size of the clustering; the number of clusters,|c|denotes the sizeofcluster c,i.e.,thenumberofdataobjectsinthecluster.A cluster is not allowed to be empty:c 6= ∅ or |c| 6= 0,and the conjunction of all clusters contains all data objects of the dataset:c1 ∪c2 ∪...∪ck = X.In a non-overlapping clustering,all clusters are mutually disjunct:ci ∩ cj = ∅,for i 6= j.If the mutual disjunction criteria is relaxed,clus- tering C is said to be overlapping.Here we only consider non-overlapping clustering.The Euclidean distance metric d(xi,xj) is used to cal- culate the distance between two data objects xi and xj.d(x,c) is used to indicate the set of distances between data object x and all data objects in cluster c:d(x,c) = {d(x,x0 1),d(x,x0 2),...,d(x,x0 |c| )} with x0 ∈ c.The inner-cluster distance of cluster c is used as the basis for the fitness function and most of the heuristics,and is calculated as fol-
lows:
D(c) =
|c|−1 X l=1
|c| Xm =l+1
d(xl,xm) (1)
where xl ∈ c and xm ∈ c.If the inner-cluster distance is calculated for a clustering C,it produces a set contain- ingtheinner-clusterdistancesofallclustersintheclustering:D(C) = {D(c1),D(c2),...,D(ck)}.Theinner-clusterdis- tance of data object x is defined as its contribution to the inner-cluster distance of the cluster it belong to:D(x) = P|c| i=1 d(x,xi) where x ∈ c and xi ∈ c.If the inner-cluster distance of a dataset X is calculated,it produces a set con- taining the inner-cluster distances of all data objects in the dataset:D(X) = {D(x1),D(x2),...,D(xN)).The fitness functionusedtoevaluateclusteringCisthecumulativeinner- cluster distance of the clustering:
f(C) =
k X i=1
D(ci) (2)
where ci ∈ C.
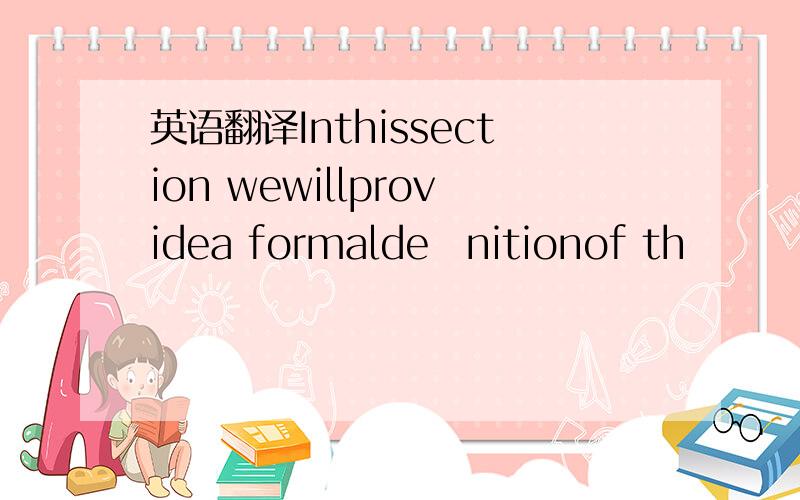
inthissection
wewillprovidea甲醛fi界定传统的聚类teringproblemandintroduceanotationusedintherestofthe纸.在超或下标的使用,我们将重用我,J,L,M没有意义是隐含在这些进行了脱fi定义之间.按照惯例在文学作品中,粗体显示设置变量,与| Y |
defi定义为埃尔-数等在Y,即,它的大小.一个n维特征或属性向量x =(X1,X2,……,XN)被称为一个数据对象,与西,(i =
1,2,……,n)的第i个特征,属性,或数据值.一个数据集X = { X1,X2,……n},具有n = | X |数据对象,与西,(i =
1,2,……,N)第i数据对象.聚类C是胶原的数据对象的子集的CI经文(我= 1,2,……,K),称为集群,K = | C
|传统保留表示大小的聚类;聚类数目,| C |表示sizeofcluster
C,即,thenumberofdataobjectsinthecluster.群集是不允许是空的:6 =∅或| C | 6 = 0,和所有的集群连接包含的数据集的所有数据对象:C1 C2∪∪……∪CK
= x.在一个非重叠的聚类,所有的集群相互间断:CI∩CJ =∅,我6 =如果相互分离的标准是轻松的,聚类等C说是重叠.在这里我们只考虑非重叠的聚类.欧氏距离度量d(西,XJ)是用来计算计算两个数据对象xi和xj之间的距离.D(X,C)是用来表示数据对象x和所有数据簇中的对象之间的距离设定:C D(X,C)= { D(x,X0 1),D(x,X0 2),……,D(x,| C | x0 X0)}∈C.集群C簇内距离为fi性功能的基础上,大部分的启发式算法,并计算如下:D(C)= | C |−1
x = 1 | C | XM = L + 1D(XL,XM)(1)在XL∈C和XM∈如果类内距离计算的聚类C,它产生了一组包含ingtheinner clusterdistancesofallclustersintheclustering:D(C)= { D(C1),D(C2),…,D(CK)}.内clusterdis -数据对象x距离是fi奈德对它属于聚类簇内距离的贡献:D(x)= P | C |我= 1
D(X,XI)X∈C和西∈如果一个集X的簇内距离的计算,它产生了一组CON组,泰宁的数据集的所有数据对象簇内距离:D(x)= {
D(X1),D(X2),……,D(XN)).该fi性functionusedtoevaluateclusteringcisthecumulativeinner -聚类簇的距离:F(C)= K x = 1D(CI)(2),CI∈C.
wewillprovidea甲醛fi界定传统的聚类teringproblemandintroduceanotationusedintherestofthe纸.在超或下标的使用,我们将重用我,J,L,M没有意义是隐含在这些进行了脱fi定义之间.按照惯例在文学作品中,粗体显示设置变量,与| Y |
defi定义为埃尔-数等在Y,即,它的大小.一个n维特征或属性向量x =(X1,X2,……,XN)被称为一个数据对象,与西,(i =
1,2,……,n)的第i个特征,属性,或数据值.一个数据集X = { X1,X2,……n},具有n = | X |数据对象,与西,(i =
1,2,……,N)第i数据对象.聚类C是胶原的数据对象的子集的CI经文(我= 1,2,……,K),称为集群,K = | C
|传统保留表示大小的聚类;聚类数目,| C |表示sizeofcluster
C,即,thenumberofdataobjectsinthecluster.群集是不允许是空的:6 =∅或| C | 6 = 0,和所有的集群连接包含的数据集的所有数据对象:C1 C2∪∪……∪CK
= x.在一个非重叠的聚类,所有的集群相互间断:CI∩CJ =∅,我6 =如果相互分离的标准是轻松的,聚类等C说是重叠.在这里我们只考虑非重叠的聚类.欧氏距离度量d(西,XJ)是用来计算计算两个数据对象xi和xj之间的距离.D(X,C)是用来表示数据对象x和所有数据簇中的对象之间的距离设定:C D(X,C)= { D(x,X0 1),D(x,X0 2),……,D(x,| C | x0 X0)}∈C.集群C簇内距离为fi性功能的基础上,大部分的启发式算法,并计算如下:D(C)= | C |−1
x = 1 | C | XM = L + 1D(XL,XM)(1)在XL∈C和XM∈如果类内距离计算的聚类C,它产生了一组包含ingtheinner clusterdistancesofallclustersintheclustering:D(C)= { D(C1),D(C2),…,D(CK)}.内clusterdis -数据对象x距离是fi奈德对它属于聚类簇内距离的贡献:D(x)= P | C |我= 1
D(X,XI)X∈C和西∈如果一个集X的簇内距离的计算,它产生了一组CON组,泰宁的数据集的所有数据对象簇内距离:D(x)= {
D(X1),D(X2),……,D(XN)).该fi性functionusedtoevaluateclusteringcisthecumulativeinner -聚类簇的距离:F(C)= K x = 1D(CI)(2),CI∈C.
英语翻译-------------------------------- go home ------------.Th
英语翻译Valuation involves a rigorously defined process th
英语翻译ʃʒ:thʒ:
英语翻译There’snolovelikeyourlove,andnoothercouldgivemorelove,Th
英语翻译To paraphrase 18th-century statesman EdmundBurke,“all th
英语翻译100th,1000th,1000000th,19243rd,1000000,1000000000,1875,1
英语翻译我不大理解10th 拜谢!
英语翻译th 和 rd 还有什么?
英语翻译the 16th President Lincoln.急
英语翻译原句是:on april 12th ,1961
英语翻译the 99th percentile events急用
英语翻译waterfront revitalization:stretching invitingly along th